A Brief Overview Of The Role Of AI In Retail
Valued at $10.66 billion (USD) in 2024, the market size of AI in retail is estimated to touch $105.59 billion by 2032 at 33.2% CAGR during the forecast period.
This staggering growth reveals a momentous drift in the conventional retail format to smarter shopping with AI; steadily progressing from ‘just selling’ to connecting with customers at a deeper and more meaningful level for lasting brand relationships.
Consumers these days expect more than just transactions. They seek efficient shopping journeys that are tailored to their individual preferences, topped with seamless payment options, integrated access to loyalty benefits across all sales touchpoints, personalized offers and most importantly, they need to feel valued by the brands through dedicated communications.
AI enables retailers to achieve this by providing the tools to understand customer preferences at a granular level. This deep understanding allows highly relevant product recommendations to create tailored experiences that cater to the unique needs and tastes of every individual shopper. The focus on ‘smarter’ experiences underscores customer-centricity, where AI serves as the essential technology for amplified CX and CLV.
As far as brands are concerned, AI in retail enables sellers to optimize their operations, predict emerging trends accurately and streamline their processes accordingly, enabling smarter experience in brand interactions while facilitating cost-effective business processes.
Furthermore, retailers also leverage AI to enhance content creation, generate personalized marketing materials, and offer 24/7 customer support via chatbot or virtual assistant to transform frontline operations. The increasing adoption of predictive and prescriptive AI is enabling businesses to improve critical functions such as demand forecasting, inventory management, and overall supply chain optimization, aiding competitive predominance in the market.
At the moment, over 87% of retailers have already deployed AI in at least one aspect of their operations. 72% of them have experienced a significant reduction in their operational costs, while 69% have reported an increase in their annual revenue.
In this blog we will discuss 7 AI use cases that are driving retail transformation, and illustrating how smarter shopping with AI is becoming the new standard in the retail world.
Top 7 AI Use Cases for Smarter Shopping Experiences
AI use cases in retail is extensive, and covers everything from creating distinctive shopping experiences to predicting demand, description generation, tagged promotions, integrated tailored loyalty programs and many more. However, there are 7 use cases that out shine all others in the realm of retail for enabling smarter shopping with AI.
- Personalized Product Recommendations –
76% lose faith in brands that don’t offer personalized product suggestions.
In a loyalty-based shopping environment, non-personalization is a potential business risk. Therefore, holding on to rule-based non-AI recommendation systems could only lead to lost customers and an emaciated brand identity.
AI powered recommendation systems on the other hand, use Machine Learning (ML) techniques like Collaborative Filtering, Content Filtering and Hybrid Models delving into vast quantities of customer data, including their purchase history, browsing behavior, items in cart, demographic information and even their interactions on social media platforms, to intricately identify purchase patterns and accurately predict what they may buy next. The suggestions are offered accordingly.
The result? Enhanced CX through quick, efficient and seamless shopping followed by increased sale, revenue and brand image for the sellers.
Did you know, Amazon gets 35% of sales from its personalized recommendation algorithm?
From individually customized home pages to ‘customers who bought this also bought…’ feature, Amazon prioritizes personalization among other key AI use cases, using mostly generative AI to refine recommendations and optimize descriptions to reach target customers and influence their purchase decisions.
In fact, Amazon has democratized its AI based recommendation service called ‘Amazon Personalize’, allowing other businesses to build and deploy Amazon’s AI-powered recommendation engines.
- Chatbots and Virtual Assistants
90% of businesses have experienced faster resolutions of issues with chatbots. Company leaders have further expressed that using chatbots has helped increase customer support satisfaction scores by 24% (approx)
Chatbots and virtual assistants are indispensable use cases of AI in retail. They facilitate 24/7 support so that customers can receive immediate responses to their queries and have their issues resolved at any time of the day. Even though they are in a stage of improvement, they are capable of handling critical tasks, like answering product related questions, providing helpful shopping tips, assisting with crucial processes like order tracking, managing returns etc.
With NLP-powered conversational AI at its core, these chatbots can understand and interpret customer queries expressed in natural human language and respond in a manner that feels intuitive and human-like.
Did you know that H&M’s Kik Chabot achieved a staggering 86% engagement rate, averaging 4 minutes of interaction per user?
Leveraging NLP to intricately comprehend shoppers’ needs, H&M’s Kik chatbot provides personalized styling tips, product recommendations, personality quizzes, and easy checkouts. The outcomes of deploying Kik were outstanding, with heavily increased customer engagement, improved shopping experiences, and higher conversion rates, posing a striking example of AI use cases in shopping.
Similarly, Pizza Hut’s chatbot contributed to 60% of its order volume and currently holds a 4.5-star rating in Facebook Messenger with over 100,000 user interactions per month.
- Visual Search and Image Recognition –
Did you know, search abandonment causes businesses to lose $ 2 trillion (USD) every year, with 68% turning away from shopping sites owing to poor search experiences?
74% of customers have admitted that they have never found the right products with text-based keyword searches, making it a tedious and inefficient process.
With potent technologies like image recognition, computer vision, deep learning, neural networks and NLP, visual search and image recognition can generate quick, relevant and intuitive search results.
The holy grail for shoppers who aren’t as expressive with words, visual search allows customers to upload pictures from the real world or screenshots from the digital world to find the same product or identical products that match the colour, texture, style, prints, patterns and price, with unbelievable accuracy.
Today 62% of millennials prefer visual search over conventional search methods. As far as sellers are concerned, brands that use features like ‘search the look’ have experienced about 20% increase in their order volumes.
- Inventory Management and Demand Forecasting –
Stock-outs can cost retailers more than $1 trillion (USD) every year with 37% of shoppers switching to another brand after the first stock-out experience and 70% on their 3rd stock-out experience.
Stock-outs damage customer experiences. Unavailability of products set in subscription mode or ordering products only to face a cancellation soon after (overselling) can lead to lost customers, lost revenues and a shrivelled brand image.
By employing AI in retail inventory management and demand forecasting, the problem of over- and understocking can be nipped in the bud. AI tools for demand forecasting help sellers with –
- An accurate estimate of the demand expectancy for a certain product in a certain season
- The optimum stock level they should maintain in their warehouses
- automated low-stock alerts to facilitate timely replenishments
- unification of inventory across all platforms of sale, facilitating smarter shopping with AI.
- Reorder-point setting
- ABC Analysis implementation to grade stock based on its performance
- Automated audits, and more.
- Dynamic Pricing Strategies –
Sellers can increase their profit margins by almost 10% with dynamic pricing.
Dynamic pricing is all about pricing fluidity defying the conventional MRPs based on manufacturing and supply chain costs. It is more about positioning products at the right place, at the right time to the right customers at an optimal price that is based on factors like current demand, competitor pricing, existing inventory levels, seasonal trends and even individual customer behavior.
AI in retail pricing overcomes barriers of manual pricing with the help of intensive Machine Learning algorithms, which process vast datasets to identify patterns and predict trends. This allows retailers to set price points that help
- maximize profitability
- Maintain competitive market relevance
- clear slow slow-moving stock
- prevent stock wastage and
- free warehouse spaces
Dynamic pricing for customers translates into advantages such as more opportunities for personalized discounts/promotions and lower prices during lean hours/seasons.
Common examples of dynamic pricing are airline bookings and reservations, hotels and B&Bs, ride-booking services like Uber, and many more.
- In-Store Experiences Enhancement –
58% of customers still prefer to shop from physical stores, and 75% are sure to make a purchase if they receive excellent in-store services.
Crafting futuristically aligned in-store experiences can transform any brick-and-mortar sales point into profitable arms for omnichannel selling. One of the most potent ways to achieve this is by facilitating smarter shopping with AI that includes convenience-based innovations like –
- Cashier-less checkouts – Here, a combination of advanced technologies, including computer vision, sensor fusion and deep learning, are used to track the products selected by customers as they move through the store, then automatically register the items in their virtual cart and charge their linked account when they leave the store. This effectively reduces wait times by eliminating conventional checkout lines.
- AI-integrated smart mirrors—Placed in fitting rooms and sales floors, these mirrors allow customers to try on clothing and accessories virtually, explore different styles and colours, and even receive personalized styling recommendations based on their preferences and body type. They also provide detailed product information, facilitate seamless payment options, and offer upgraded delivery and return services, further enriching in-store interactions.
Amazon Go Store is a classic example of AI use case in shopping in-store with cashier-less checkouts. Similarly, Sephora helps beauty enthusiasts find the perfect makeup shades without having to actually try them on, with their virtual mirrors.
- Fraud Detection and Secure Transactions –
Transaction frauds cause e-commerce businesses to lose $48 billion every year.
Both customers and sellers expect a safe, secure and scam-free transaction environment. AI fraud prevention systems, in this respect, uses sophisticated algorithms like ML and predictive analytics to pinpoint suspicious transaction patterns and anomalies that may indicate potential fraud.
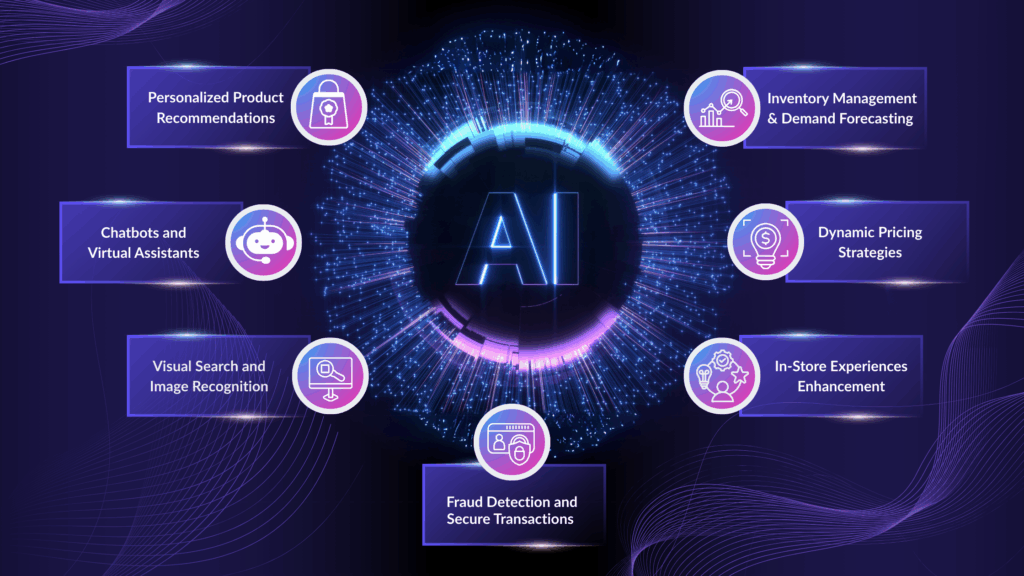
AI fraud prevention tools monitor critical aspects of a transaction, which include spending patterns, login attempts and shipping information, to identify potentially risky devices or behaviors. This prevents unauthorized transactions and safeguards sensitive customer data. Be it credit card fraud, return fraud and even employee theft, AI in retail helps sellers minimize financial losses and maintain the integrity of their operations.
The Future of Smarter Shopping with AI – Trends To Look Out For
Artificial Intelligence in the retail industry is not a fleeting trend. It is projected to grow and evolve with the passing years to facilitate smarter shopping with AI upcoming innovations like emotion awareness recommendations, voice-enabled assistants, predictive customer engagement, glance AI and more.
Some of the emerging trends of AI in retail that have been taking online and offline selling by storm include Generative AI for evolved hyper-personalized shopping, Agentic AI to automate complex operational tasks like inventory management, Multimodal AI for nuanced understanding of customer preferences and AI-powered sustainability to make more environmentally conscious decisions.
Final Thoughts – As they say, the future is now, and it keeps getting better with AI. Seven key AI use cases, from personalized recommendations to fraud detection, are creating smarter, more convenient shopping experiences, driving retail innovation to new heights. With this transformative disruption, one can say, the journey of AI use cases in shopping has just begun, and the world of retail is positioned to explore surprising opportunities in the days to come.